Daedalean Concluded a Joint Research Project with the FAA on Neural Network-Based Runway Landing Guidance for General Aviation
On May 23, 2022, the FAA William J. Hughes Technical Center Aviation Research Division Atlantic City International Airport made publicly available on the FAA website a 140-page report titled “Neural Network Based Runway Landing Guidance for General Aviation Autoland”.
This report is the primary outcome of a joint research project between the Federal Aviation Administration and Daedalean, a leader in developing certifiable aviation-grade applications based on neural networks and machine learning. The subject of the project was the study of a visual landing system (VLS) for fixed-wing aircraft developed by Daedalean.
The system is based on Machine Learning (ML) or what is often referred to as “Artificial Intelligence.” The flight test campaign took place in March 2021 in Florida. It was conducted in the presence of FAA members on board. The test aircraft was provided by Avidyne Corporation, a partner of Daedalean in the development of the first ML-based airborne systems for General Aviation (GA).
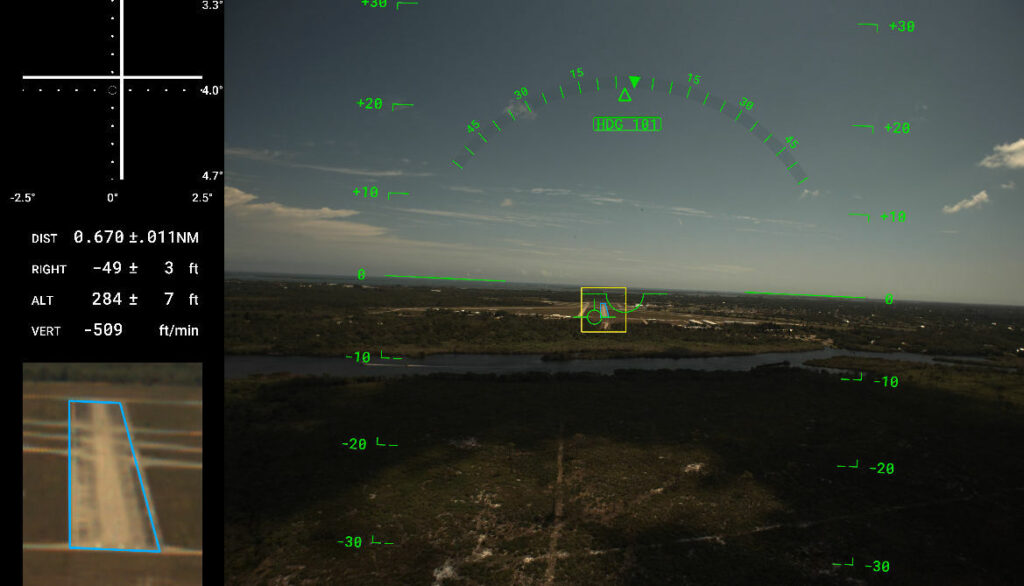
The project had two goals: first, evaluating whether the W-shaped Learning Assurance process can satisfy the FAA’s intent for setting the future certification policy; and second, assessing a visual-based AI landing assistance as a backup for other navigation systems on a low-risk first implementation of AI-based systems.
Evaluation of NN-Based Technology: Landing Assistance in 14 CFR Part 91 GA Aircraft
The particular goal here was to validate whether a visual-based AI landing assistance can serve as a backup for other navigation systems in case of a GPS outage, on a use case where it was implemented first for General Aviation as Non-Required Safety Enhancing Equipment (NORSEE).
Dr. Luuk van Dijk, CEO & Founder of Daedalean, said:Visual landing is one of the building bricks for the future full autonomy; but today, VLS is aimed at serving as pilot assistance on fixed-wing aircraft and rotorcraft. During the test flights, we flew standard landings and robustness tests of our systems, with two flights and eighteen approaches, both over previously trained and untrained runways, in various conditions, including degraded daylights, steep glideslope angles, and sharp roll manoeuvres. The system performed well; in particular, it spotted a runway that was not part of the training set from almost 5 km (3 NM).
Researching the Compatibility of the Learning Assurance Process with the FAA Regulatory Framework
The scope of this study was to test in practice the W-shaped Learning Assurance process to verify it can satisfy the intent of the FAA certification and development assurance processes. The W-shaped Learning Assurance process was the outcome of the two joint Concepts of Assurance for Design of Neural Networks (CoDANN) reports (CoDANN20; CoDANN21) by the European Aviation Safety Agency (EASA) and Daedalean.
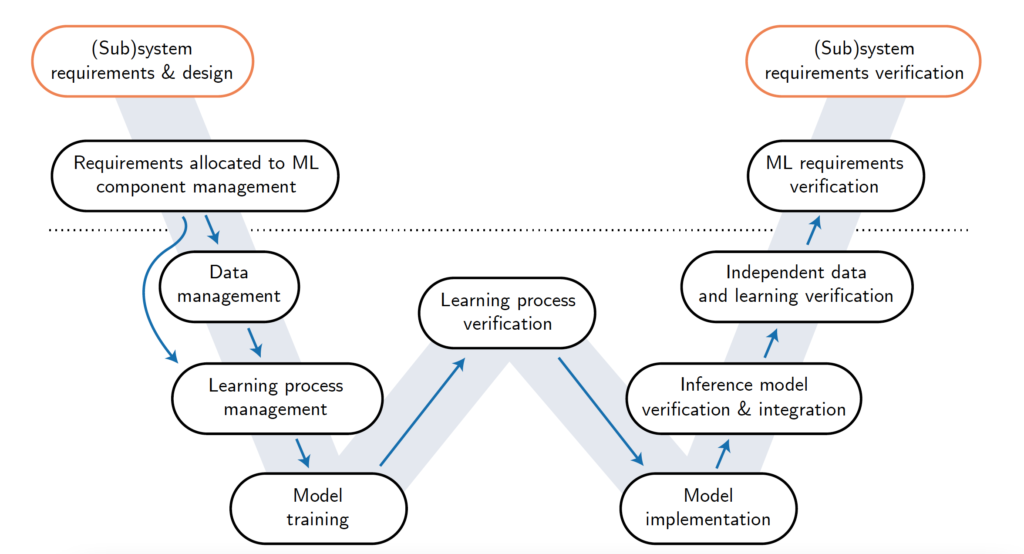
This way, the FAA could gain experience with machine learning/neural network-based applications, using a specific example (landing guidance) proposed by Daedalean in order to inform on the specific policy for ML-/NN-based systems, future certification requirements, and future industry standards for AI/NN NORSEE systems. The report further states that the results of the research may be used by the FAA for certification policy development, in particular regarding the reliability, robustness, and real-world capability of such systems.
Dr. Corentin Perret-Gentil, head of Daedalean’s ML-research group, said:In the report, we provided a detailed walk-through on the design and evaluation of a machine learning-based system targeted to safety-critical applications. We showed how the W-shaped process provides elements for a thorough safety assessment and performance guarantees of an ML system. We demonstrated how this is done by exploring data requirements, generalization of neural networks, out-of-distribution detection, and integrating traditional filtering and tracking.
See more about the test flights conducted during the project on Daedalean’s blog post describing testing the performance of the system under various visual conditions, and watch the video.
This article was originally published by Daedalean.