Waymo is continuing to invest in understanding weather and its impact on autonomous driving.
Weather can impact the Waymo Driver system in several ways:
- Wet roads can create reflections that are perceived as objects by the cameras
- Condensation can alter sensor data
- Droplets, dirt and ice can impair the surface of sensors
- Wet and icy roads can be slippery, reducing friction
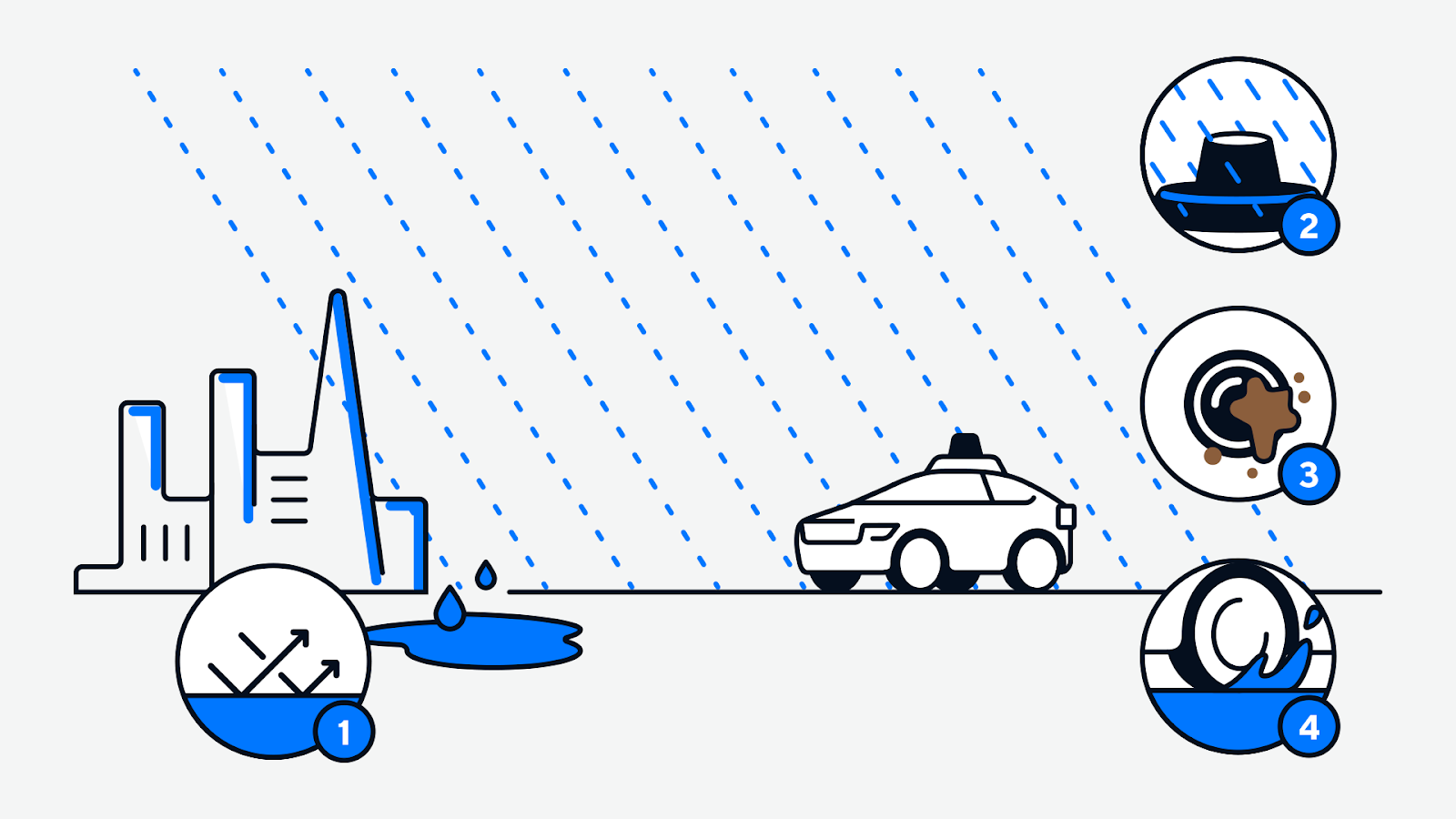
To combat these challenges, Waymo’s latest hardware uses the presence of raindrops on its windows to classify various weather conditions.
In addition, it uses laser-based instruments to measure the properties of clouds, fog, dust storms, snow and rainfall, alike to those use by weather researchers.
This data is combined with high-quality ground-truth data from weather visibility sensors to generate a quantitative metric about meteorological visibility.
This metric enables the Waymo Driver to conduct a quantitative analysis of the weather in real-time, operating as a ‘mobile weather station.’
Weather Maps
Existing weather stations are often not located in areas where cars drive, causing the data to lack the precision and specificity that reflects real-world conditions.
Indeed, weather stations are typically located at airports, where conditions can vary significantly compared to surrounding neighbourhoods.
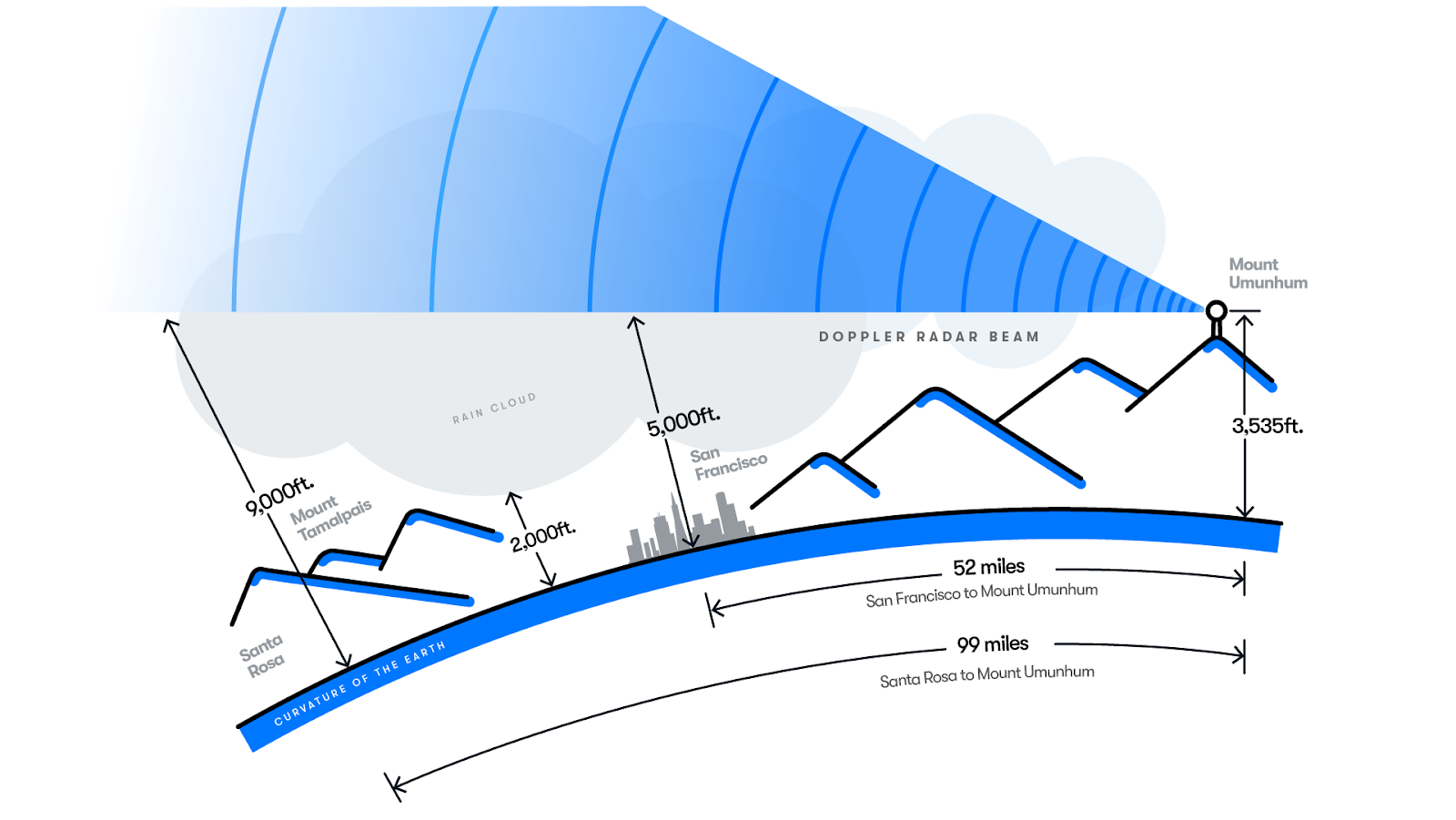
While remotely sensed weather data from satellites and radars can also contribute to weather data, they do not directly sense conditions near the surface, especially when conditions are cloudy.
In response to these inaccuracies, Waymo is using its autonomous vehicles to act as ‘mobile weather stations,’ enabling the company to develop a first-of-its-kind fog map that can better inform its operations.
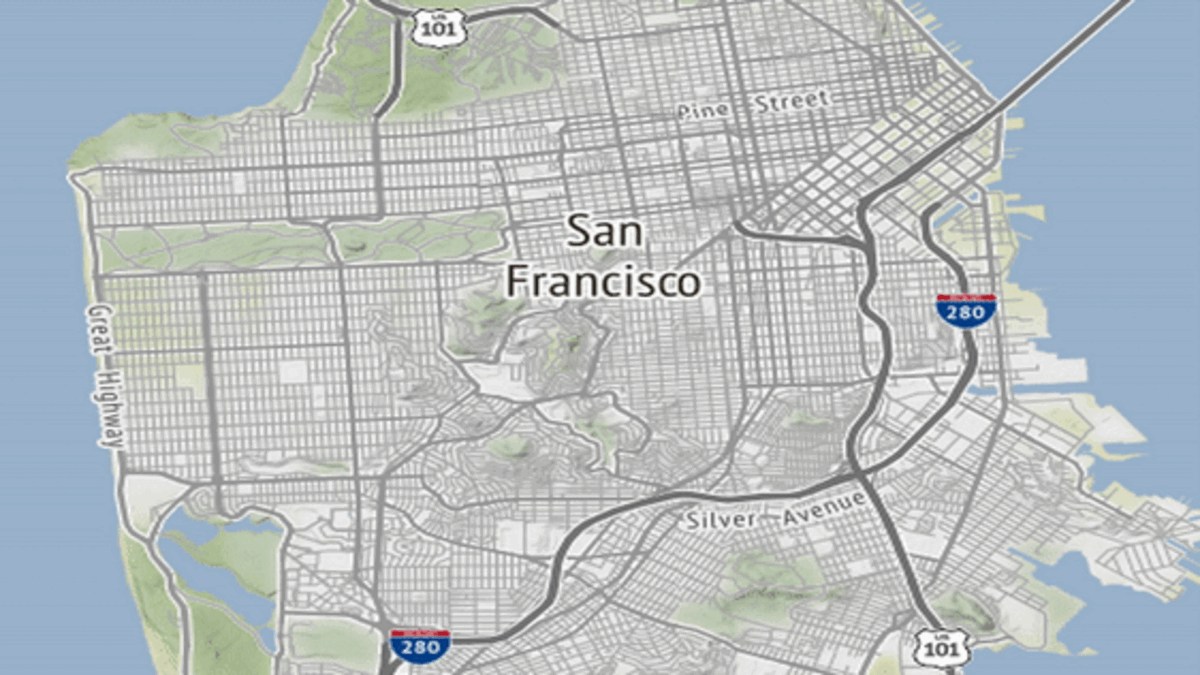
With these insights, Waymo’s autonomous fleet can track the progression of weather such as coastal fogs. In addition, it can detect drizzle and light rain that lead to wet roads in situations that are invisible to the National Weather Service’s local Doppler weather radar.
These observation capabilities allow Waymo to localise weather conditions and determine where they are beginning to deteriorate or improve. This information can then be used to optimise the performance of its autonomous vehicles.